Big Data and Your Future as a Data Scientist
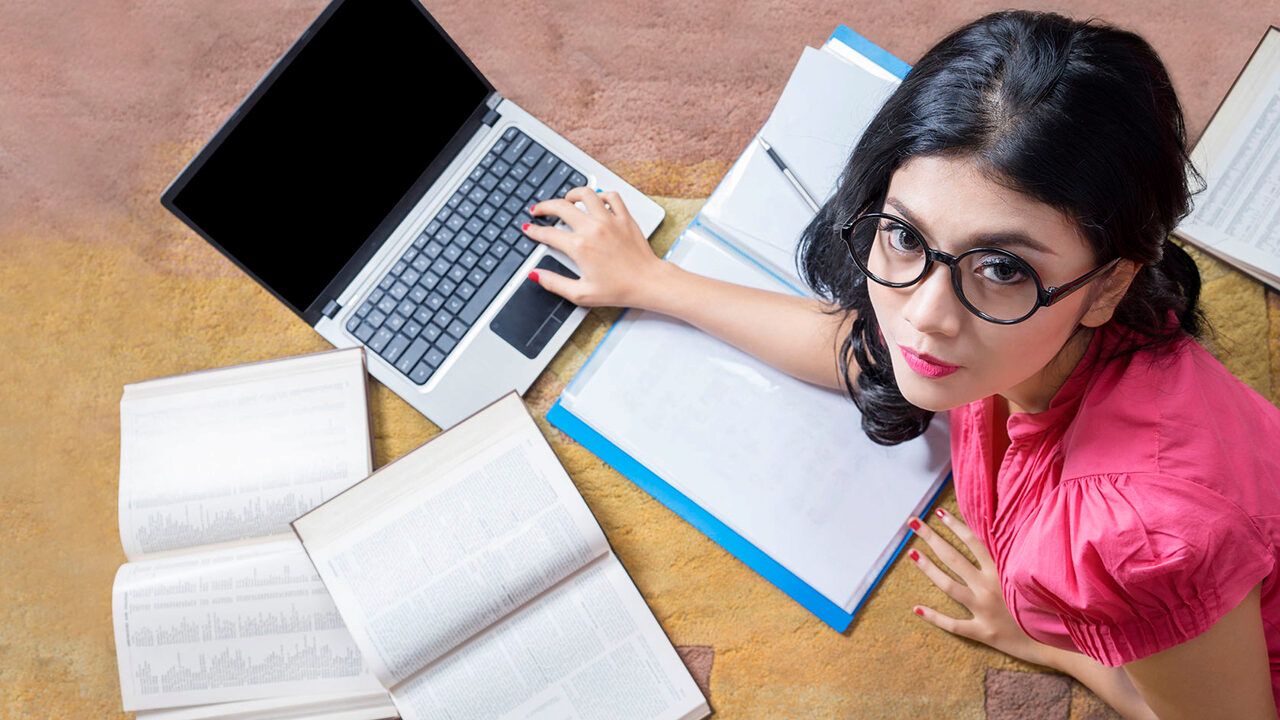
Have you heard of the Big Data Challenge? Run by the STEM Fellowship, it’s a competition that helps high school students get excited about data science. This year’s theme: Think Global and Act Local with Big Data.
While big data is the darling of some Science, Technology, Engineering and Mathematics programs, most mainstream high school students have no idea what it is or how it’s transforming the business world – and your opportunities for employment. While the industry has only existed for a decade, big data is everywhere. It has spread across the corporate landscape like wildfire in a blaze of statistics, analysis, and new titles like vice president of big data and chief data architect.
Forbes offers a simple definition sound bite: “Big data is a collection of data from traditional and digital sources inside and outside a company that represents a source for ongoing discovery and analysis.” In other words, the business world collects and examines big data to operate more efficiently and effectively in ways like saving money and investing it wisely in new products and services, improving its customer base and relationships, gaining competitive advantage over other companies, and, ideally, becoming more successful. The job description for a recently created position, vice president of customer insights and operational excellence, is to use big data analytics to understand customers, develop new products and cut operational costs.
KWHS spoke with Wharton marketing professor Peter Fader, an expert in data analysis, to help us understand the mysteries locked in all these numbers, and what they mean for tomorrow’s job prospects.
KWHS: What is big data?
Peter Fader: Let’s first talk about what big data isn’t. Too often people hear those words and they immediately think about the sheer volume of the data – more specifically, how many different customers we’re looking at or how many rows are in our database. Volume is part of it. But most of the action in big data is not in the rows or the number of customers you have, it’s in the different measures you have for each customer. In the old days, all we knew about customers was their demographics. You could look at someone and see that he was a 56-year-old white male and you would put him in a bucket. That was all you had. In the 1970s, we started to track behavior so we would know which soup you were buying and connect it to other purchases within the grocery store. And then in the 1980s and 1990s, we started building CRM systems, customer relationship management, that would let us connect different kinds of purchases. We could look at your purchases in a grocery store and connect them with your purchases in a department store. Or, we might be able to connect it with your media exposure so that we knew what advertisements you saw and what stuff you bought. This is the birth of big data. When you’re looking at seemingly unrelated data sources and connecting them together at a granular level.
Now let’s fast forward to where we are today. Not only do we know a lot about the customers, but we also know things like geolocation – where the customer was when he took a certain action. Things like biometrics – what was your heart rate when you purchased something? There’s an emerging field of neuroscience – what parts of the brain light up when you do certain things. There’s social networking – not only what I’m doing, but what people closely connected to me are doing. There’s social media – what are people saying to and about each other. It’s all these different fields ideally linked together at the customer level, and trying to get greater insight out of any one of these fields to be able to answer really deep questions about not only who is going to buy what next, but why. In theory, it gets us to a much better understanding of the overall customer experience or journey than what would have been conceivable a few years ago. That’s big data.
KWHS: How does big data relate to data analytics?
Fader: Having a better grip on who is buying what when in order to forecast the expense of new products or new marketing campaigns begins to get at why we need big data. But in order to do so, we have to get below the surface of the data itself. The data by itself doesn’t answer what’s happening in the future or what’s happening below the surface. That’s where the analytics come in. You can collect all the data you want and have a good time doing data science, just kind of mucking around with the data and seeing what correlates with what. You really need to get past the raw data and either project what the future data’s going to look like or get below the data to start asking questions about the true, underlying, unobservable propensities that generate the data. That’s the stuff that companies really need. That’s where analytics really shines – being able to go beyond the raw data.
KWHS: What are the job prospects related to the field of big data?
Fader: There are a ton of jobs purely on the data-collection side. Developing technologies that are either aimed at collecting and creating the big data structure, or that do so as a side benefit of some other thing. Walmart has a new program called Scan and Go. It’s a mobile app. You walk into Walmart and you basically scan your purchases yourself. You pull out your phone, scan each product with your phone, and when you go to check out instead of scanning each item in your basket, you hold up your phone with one code and it shows the cashier all the things you bought at once. Push a button and you’re done. Walmart is doing this because it’s a quicker, easier shopping experience and they pay less in labor. Along the way, they’re collecting all this cool data. They need to hire people who will help them manage it, and they need to hire people who will help them leverage it. So that’s one area of employment: hiring people to do data collection or to manage the information that arises from leading-edge data collection tasks.
That leads to the next step. We have each of these different interesting new data structures coming in, so how do we get it all to link together at the household level? That’s where data science really shines. That ability to manage and merge and dedupe [data deduplication], which means looking at duplicate records from households and combining them together. There are all kinds of technical and soft skills involved in putting the data in a form that will not only make it clean and neat, but will also enhance the ability to do analysis on it.
That takes us to the next step, which is doing the analysis on the data. I think data management is really important and will be a lucrative field for many smart people. Data science is the next step. It’s about getting the science from the data set. How do we forecast or get below the surface to explain why. That’s science. It requires a scientific process of asking hypotheses and knowing how to test them. Or building statistical models that will help us take the data in directions that the raw data don’t necessarily thoroughly answer. The deeper analysis is data science.
The next step would be enabling decision-making. Yes, it’s great to be able to make this forecast and to get underlying insights about who is buying what and why, but why are we doing this? Because companies want to make more money. They want to develop better products. They want to acquire better customers. Being able to turn those analyses into action requires a beautiful blend of scientific and business skills. It used to be that people could do great at business without having that analytical angle. In fact, being analytical would actually get in the way and lead to analysis paralysis. Today, you need to have both skill sets to ask the questions, know how to answer them, and then to take action on them. Today’s managers require a different set of skills.
KWHS: Are high school students prepared for the big data economy?
Fader: A lot of these skills and the technologies required to do them didn’t exist 10 years ago, so there’s just no room for them in the high school curriculum. A student’s day is already full and the school’s faculty is already staffed up. Where are we going to fit in that course on data management? How are we going to afford to hire another teacher to teach that stuff? In many cases, these things get crowded out from a high school education because there’s no room for them. A lot of people say we should allow programming languages like Python to be emphasized and required as much as foreign languages. It’s important to have this kind of conversation about what students need to learn.
If you look at the math curriculum, the way we’re teaching math today: algebra, geometry and so on, is the same as it ever was. Very few students are taking statistics in high school, and if they are it’s a tack-on to the end. It’s really left to the students or their parents to do an extracurricular like programming camp [to prepare students for careers in areas like big data]. This also leads to the great digital divide. Parents with money and time can sign junior up for these courses, but there are a lot of really smart people who don’t get exposure to these classes.
If you only have limited amounts of math to teach, especially for kids who may not go to college or have limited math once they’re there, we may want to rethink the types of courses that are offered in high school. Students would be better off learning more about probabilities and statistics. We are teaching them a lot of stuff in college that they should be learning in high school. A great example is Microsoft Excel. Everyone should be learning this in high school. You should not be able to graduate from high school without being reasonably fluent in Excel. The fact that we have to teach Excel 101 and programming 101 and probability and statistics 101 means that half your college time is gone before you can start getting in deep and exploring some of these skills. Even at a top school, we are not seeing students who are ready to hit the ground running as data scientists.
KWHS: What fuels your personal interest in big data and data analytics?
Fader: I’ve always been interested in forecasting things even when I was in high school, whether it was sports or music. Let’s look at the billboard charts and predict what song will be No. 1 next week. It’s a fun game. Anyone who is playing fantasy football or anything else in the forecasting business knows this. Part of it is a desire to do that well. It’s the process of saying how many factors do I need to take into account? How complex do I have to make it, but not too complex that the forecasts go haywire? It’s about finding that just-right balance. Students should familiarize themselves with the concept of Occam’s Razor [the process of paring down information to make it easier to find the truth]. The whole idea is that the best explanation or best forecast will be the simplest, plausible one. We can easily go out there and complicate things, but the more you overcomplicate, the worse your forecast is going to get. Occam’s Razor is about striking that just-right balance between an explanation that’s adequately good to help us trust the forecast, but not overdoing it.
KWHS: Any advice for high school students who are interested in big data?
Fader: Too many people, because of the misnomer of data science, think that if I can just crunch those numbers, sort them and manage them, great things will happen. The big payoff in data management is in the extracting beyond the data – the forecasting and the analytics. It’s more than just data-management skills; it’s the analytical skills to frame up the right questions. Because the data are getting so big and messy, sometimes people are crowding out those analytical things because they’re having such a good time mucking around with the data. Finding the balance there is important.
Also, to the extent that our students in high school are getting outside the pure math courses and taking steps in this big data direction, very often they are doing it through economics courses. I’m not at all saying that learning economics is bad, but too often economic thinking doesn’t do justice to all the underlying data. It rests on a lot of assumptions: If people were rational, if markets were efficient, then what would happen. It’s a fun exercise to think about that, but it’s not directly aligned with probability and statistics where we’re not trying to impose a lot of assumptions, we’re trying to learn the truth. Students who want to learn about leveraging data often start doing a lot of econ stuff. I would prefer that they put probability and statistics on an equal footing.
What is big data and why is the industry so valuable to the business world?
What are three jobs related to big data and the possible skills necessary to do them well?
What is Occam’s Razor and why is this concept so critical to the field of data science?
As a big data freak, I completely agree with a lot a of the fantastic points made in this article. It definitely requires a holistic approach to thinking and problem-solving, and it’s almost a four-dimensional way of attacking and solving a problem. As someone who is highly motivated to optimize things and deconstruct the complex workings out there, I’m glad I discovered data science and big data – it’s definitely a challenging yet encouraging environment that fastens my thinking cap as tight as can possibly be.
To any high school students out there – this will be the next big thing, hands down. Big data tells us so many stories, and it tells us those stories in its own language – mathematics. To be able to decode and explain in simple English what billions of these unique data points are saying about our society is a task that takes great skill and perseverance to develop. As a beginner like myself, it can be absolutely frustrating at times to analyze data in the wrong way – trust me! This field, however, is soon to become a powerful weapon that’s not only in the hands of businesses, but whoever takes the time and energy necessary to be proficient in such a demanding industry. The sky’s the limit in big data, and how you interact with data is absolutely essential to your success. Thanks Prof. Fader for these fantastic insights!
Thank you for your thoughtful comments, Aneesh. Your personal essay published on KWHS is a perfect companion to Prof. Fader’s big-data discussion! https://globalyouth.wharton.upenn.edu/2017/09/vectors-computer-code-valuable-experience-rocket-scientist-statistics/
As a lover of technology, I might say that who administrate Big Data can control everything; the volume of information can lead to power. It’s really interesting how business can manage the data, the quantity doesn’t matter. It’s what organizations do with the data that matters. Big Data can be analyzed for insights that lead to better decisions and strategic business moves. As professor Peter Fader said, the analysis of the Big Data allow business to produce forecasts by using demographic information and other similar sets. Business can really produce high results when it comes to Big Data and data analysis.
When it comes to jobs in the area, we can find a diversity of them, such as App development, when you create an app that will collect data about people, products, money or anything! Any job related to the collection and management of data will take place on the Big Data. The set of jobs also includes data deduplication & management, and the analysis of the data. To engage and be successful in the Big Data job areas it’s essential knowledge in Statistics and Excel.
I also agree with the importance of Occam’s Razor, because it’s essential to split down information to make it easier to find the real reason behind it. “Occam’s Razor is about striking that just-right balance between an explanation that’s adequately good to help us trust the forecast, but not overdoing it”, Fader said. Therefore, the act of splitting or removing unnecessary information will show what is inside the Big Data.
Big data encompasses all fields of data management and analysis. It goes beyond sorting data and making correlations between; it requires in-depth analysis.
Analyzing big data often helps apply it to the real world. Large corporations use it to find out why consumers are buying certain products, and make adjustments accordingly.
Big data is very interesting to me. I think I should work on my programming skills and take some probability and statistics courses next year or over the summer to become better prepared to grasp big data. Regardless of what career I choose, being skilled at data management can greatly assist me in my endeavors.
Big data is the interpretation of statistics drawn from the analysis of certain studies and inquiries. It can be applied in all fields of research, including but not limited to science, demographics, and politics. This is useful in the business world because it can display supply and demand in certain industries, customer satisfaction, and the buying habits of certain demographics.
The most basic form of employment relating to big data is the collection and organization of statistics, which includes the assortment of related versus unrelated data. There are jobs dealing with the analysis and interpretation of big data. These jobs essentially involve understanding the connection that data has to the real world as well as the phenomena the aforementioned statistics suggest. Finally, there are administrative positions in which executive decisions are made based on big data.
Occam’s Razor is a concept that establishes the presentation of information, including but not limited to big data. It establishes that the most effective way to present information is in a manner that doesn’t overcomplicate the subject matter, but still provides sufficient data to explain a topic. This is important to data scientists because in order to explain data to a professional who does not specialize in data science, one must be able to express themselves both clearly and descriptively.
Big data is already everywhere in our life, from online ads and recommendations to every payment we make on the internet. Information leak is the foremost concern about this big data age where in the near future, our every move might be recorded. The Face Book data leak this year has definitely brought more attention to this issue. Indeed, the security systems should be reinforced; or data should be collected in a less personal way, making it hard to trace back from the data to the specific users. Either way, big data would play a bigger part in our life and we would have to take some risks in exchange for its convenience.
I think data science will eventually help people with their decision making in everyday life. Machines can be a great helper when we face dilemma in real life. For example, when we were deciding whether to go to a meeting by car or public transit, machine could help us find out the time it would take, how difficult it would be to find a parking lot, how crowded the public transit would be, the road conditions, etc. If we also provided information about how we think about our past journeys, an algorithm could be used to maximize our rating about the coming trip. If we could further provide data and our ratings about our other past events, perhaps the machine could learn what factor we valued more when making such decisions, thus a more suitable algorithm could be made. Likewise, other everyday dilemmas could be solved in this way.
But that’s not to say we would allow machines to determine our life. Machines would only offer suggestions on whether to go to a new movie as well as reasons behind it. They would give us a new perspective into ourselves – our preferences, our willingness to take risks, etc. Many times we might find it hard to explain why we did something, and machines could help us look into us more precisely and methodologically. Algorithms could extract our motives behind each behavior and inspire (also enable) us to have a deeper understanding of ourselves. As the creator, we, human beings, would never allow the machines to go beyond the scope of helping us and to replace our own thinking.
I want to become a data scientist and have done a project in anomaly detection by analyzing users’ keyboard and mouse events. Indeed, probability and the Occam’s razor concept are two of the very essential things in this work. I found that the way humans and machines make decisions are quite similar. Both rely on predictions and probabilities; the only difference is that humans cannot calculate all the exact data, so they rely on their experience or intuition. In the project, I found that if the operation patterns were shown in a video to people, they would be able to tell one user from another; but not as well as a computer, which could notice the slightest difference in the patterns with exact calculation. Also when learning a new language through reading, we could sense that some words appear more frequently after a given word; while through a similar model, a computer can extract the key words in a sentence to analyze its meaning. Thus I believe finding a good way to interpret data will still be an important part of data analysis since human experience can find a better model that cuts down the amount of calculation.