AI and You: Learn to Be the Human in the Loop
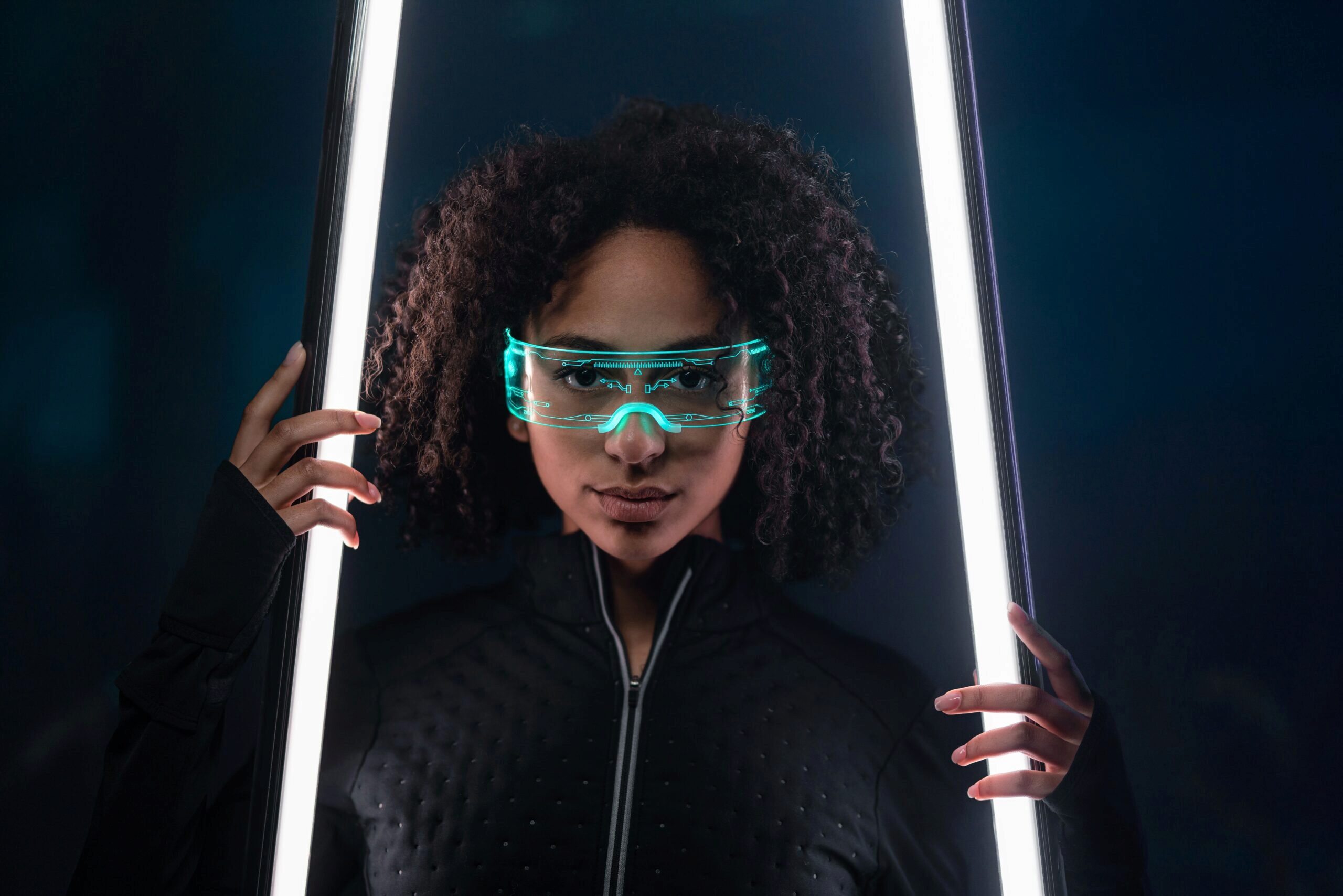
When considering the evolving human-artificial intelligence (AI) relationship, which conjunction best captures the essence of this connection? Humans AND AI or Humans OR AI?
This seems to be on lots of people’s minds as generative AI apps like ChatGPT, Bard, Dall-E2 and others become more sophisticated in generating content and images. Are machines replacing us? How do humans fit into the AI revolution? What are the most effective ways for humans to collaborate with AI systems?
The AI-Human dynamic has been a hot topic in Wharton Global Youth Program’s Cross-Program Speaker Series, during which Wharton School professors present lectures to some 300 high school students participating in summer business programs on the Wharton campus. Speakers highlight their research and engage our global youth in the latest cutting-edge business and finance topics.
That sometimes means getting a glimpse at actual business cases. Lynn Wu, a Wharton associate professor of operations, information and decisions who studies the implications and influences of technologies on business, has analyzed the human-AI dynamic in companies like DHL, a global logistics business specializing in international shipping, courier services and transportation. Her research often considers the broader context of AI (beyond ChatGPT), combining computer science and large datasets to enable problem-solving.
A Human-Augmentation Tool
According to Wu, DHL used data analytics — the process of examining data to make decisions — to improve how it shipped goods across different parts of the country and the world. The company captured data from its business processes for eight years to study the problems, and then waited three more years before using machine learning – building algorithms based on the data – to make improvements. The deep data collection and analysis were ultimately very telling about AI’s ability to advance operations.
“Having a human-machine collaboration is a new way to organize firm activities. That’s where you guys come in. You’ve got to figure out how we marry machines and humans in a new way. That is the future of our economy.” –Lynn Wu, Wharton School Professor
We shouldn’t just hand all problems off to AI, concluded Wu. “DHL found that AI systems never got it right entirely,” noted Wu. “Humans always had to monitor what was going on because machines can’t solve many of the important edge cases – things on the edge, on the border, unusual events. The edge stuff matters a lot, and machine learning is not good at edge cases. Humans had to monitor that and teach AI about how the edge cases went wrong. Through human-machine collaboration, DHL was able to significantly improve the efficiency of loading palettes onto their cargo planes and cargo trucks. Key to this process was a continuous feedback loop, where humans improved on something, AI learned from it, and then told humans what else was important.”
Wu’s research into DHL and other companies suggests that AI can improve processes but is not always ideal for radically new situations. “The core of all of it is that you’ve got to think about AI as a human-augmentation tool,” said Wu. “It’s not a replacement tool or a substitution tool. AI can do some things really well, but it can also do some things wrong. Humans need to be in the loop. And having a human-machine collaboration is a new way to organize firm activities. That’s where you guys come in. You’ve got to figure out how we marry machines and humans in a new way. That is the future of our economy.”
We Are In Control
Your job will likely get more interesting with AI, said Ethan Mollick, an associate professor of management at Wharton and academic director of Wharton Interactive. He believes that people should stop thinking about AI, in particular generative-AI technology that can answer prompts by generating full written essays and artwork and formulating complete ideas, as “overtaking” the jobs that humans have.
“I think there’s too much focus on the idea that overlap with AI means your job is gone,” said Mollick, who recorded a “Practical AI for Teachers and Students” video series on YouTube, and has written a number of articles on his Substack page to help people navigate the rapid changes brought on by ChatGPT and other generative-AI apps. “You want to be in an industry where you are heavily exposed to AI and you want to work with AI to do your job. Marketing, writing, a lot of the kinds of tasks that you guys will do are very affected [by the technology]. Professors are very affected. But that doesn’t mean my job gets worse because it’s affected. It means I get to use AI tools.”
Mollick, who spends a lot of time studying the humans AND AI landscape, encourages people to stop thinking that AI is something that is happening to them. “We have control over AI, and we get to decide how to use it,” he noted, adding that he also feels government regulation of the technology is important.
Rather than focus on “the extinction scenario” where AI takes over the world (Humans OR AI), he shared a few best practices for people to follow as we figure out how to live and work with AI:
✅ Invite AI to Everything
“As long as you’re being ethical about it, you should be using AI in everything you do.”
✅ Be the Human in the Loop
“You prompt AI best by giving it bullet points and stuff to work with. I would say, for instance, ‘write an essay with the following points,’ and then I would feed it the points. And then I would go in and say change paragraph two or I would totally rewrite it myself. You want to figure out how to become the human in the loop and work with the AI to do better things.”
✅ Tell It Who It Is (and Treat AI Like a Person)
“The biggest issue with “getting” AI seems to be the almost universal belief that, since AI is made of software, it should be treated like other software. AI is more like a person in how it operates and does a good job with writing, analysis, coding and chatting. Treat it like a person, give it feedback like a person, and teach it like a person.”
✅ This Is the Worst AI You Will Use
“Once you guys are in college or graduate college, AI will be changing almost every job. You need to figure out what that future looks like for you. When we think about jobs in the modern economic sense, we think of them as bundles of tasks. And these jobs aren’t one thing you do, they’re a lot of things you do. AI is going to take away some of those tasks — a lot of the boring parts of your job. Say you don’t like filling out expense reports. AI can do that for you. I think you are going to be handing off the worst parts of your job. I don’t need you to change your career path based on this. But you should be aware that this AI thing is real and it’s going to affect lots of jobs, especially jobs that Wharton MBAs and undergraduates tend to go into, like consulting. So, keep an eye on it.”
Conversation Starters
Professor Lynn Wu says, “The edge stuff matters a lot, and machine learning is not good at edge cases.” What does she mean by this and how does this underscore the importance of human-AI collaboration?
Do any of the insights in this article contradict what you’ve been told about AI. For instance, how do you feel about Professor Ethan Mollick’s declaration to “invite AI to everything?”
Have you had any run-ins with AI that made you question the AI-Human dynamic? Describe your experience in the comment section of this article. What conclusions did you draw from this experience and how might it inform your own future with AI?
Is coding or CS experience required to take full benefit of this summer program?
In the realm of machine learning, Professor Lynn Wu’s insight into the significance of addressing edge cases resonates deeply with my experience in a recent project on semi-supervised learning. In this project, we delved into the dynamic landscape of leveraging both labeled and unlabeled data. Unlike traditional supervised learning approaches that rely solely on labeled data, semi-supervised learning recognizes the importance of incorporating unlabeled data to enhance adaptability and performance.
This approach becomes particularly pertinent when dealing with edge cases—those instances that defy conventional categorization. By integrating unlabeled data, our semi-supervised learning model showcased a capacity to navigate and learn from scenarios that do not neatly fit into predefined categories. The synergy between labeled and unlabeled data allowed for a more nuanced understanding of complex, real-world situations, echoing the need for machine learning systems to be robust in the face of diverse and outlier-rich datasets.
In my reflections on this project, I am inclined to underscore the parallel between our semi-supervised learning strategy and the call for human-AI collaboration. While machine learning algorithms excel in pattern recognition and processing large volumes of data, the inclusion of human intuition and expertise remains pivotal, especially in scenarios where the data landscape is intricate and unconventional.
Professor Ethan Mollick’s recommendation to “invite AI to everything” resonates with the notion of harnessing AI’s strengths across diverse domains. However, my personal experience the importance of recognizing AI as a tool rather than a complete replacement for human decision-making.
In a specific instance, our team was developing a recommendation system using advanced AI algorithms for a complex project. While the AI excelled in processing vast amounts of data and identifying general patterns, it faced challenges with nuanced and context-specific scenarios. As the super semi-supervisor, my role became crucial in providing guidance, interpreting unique cases, and ensuring that the AI’s recommendations aligned with the project’s overarching goals.
This experience highlighted the need for a strategic collaboration between humans and AI. While AI can analyze data efficiently, human decision-making brings contextual understanding and the ability to navigate intricate situations. In this scenario, the super semi-supervisor role became pivotal in steering the AI towards optimal outcomes by complementing its strengths with human insight.
The key takeaway is that inviting AI to everything should be a thoughtful and strategic endeavor, recognizing the symbiotic relationship between human expertise and AI capabilities. Rather than viewing AI as a replacement, its integration should be guided by a nuanced understanding of where AI excels and where human judgment remains irreplaceable.(ISSN:0277-786X)