Analyzing F1 Motorsports Drivers in Wharton’s Moneyball FLEX
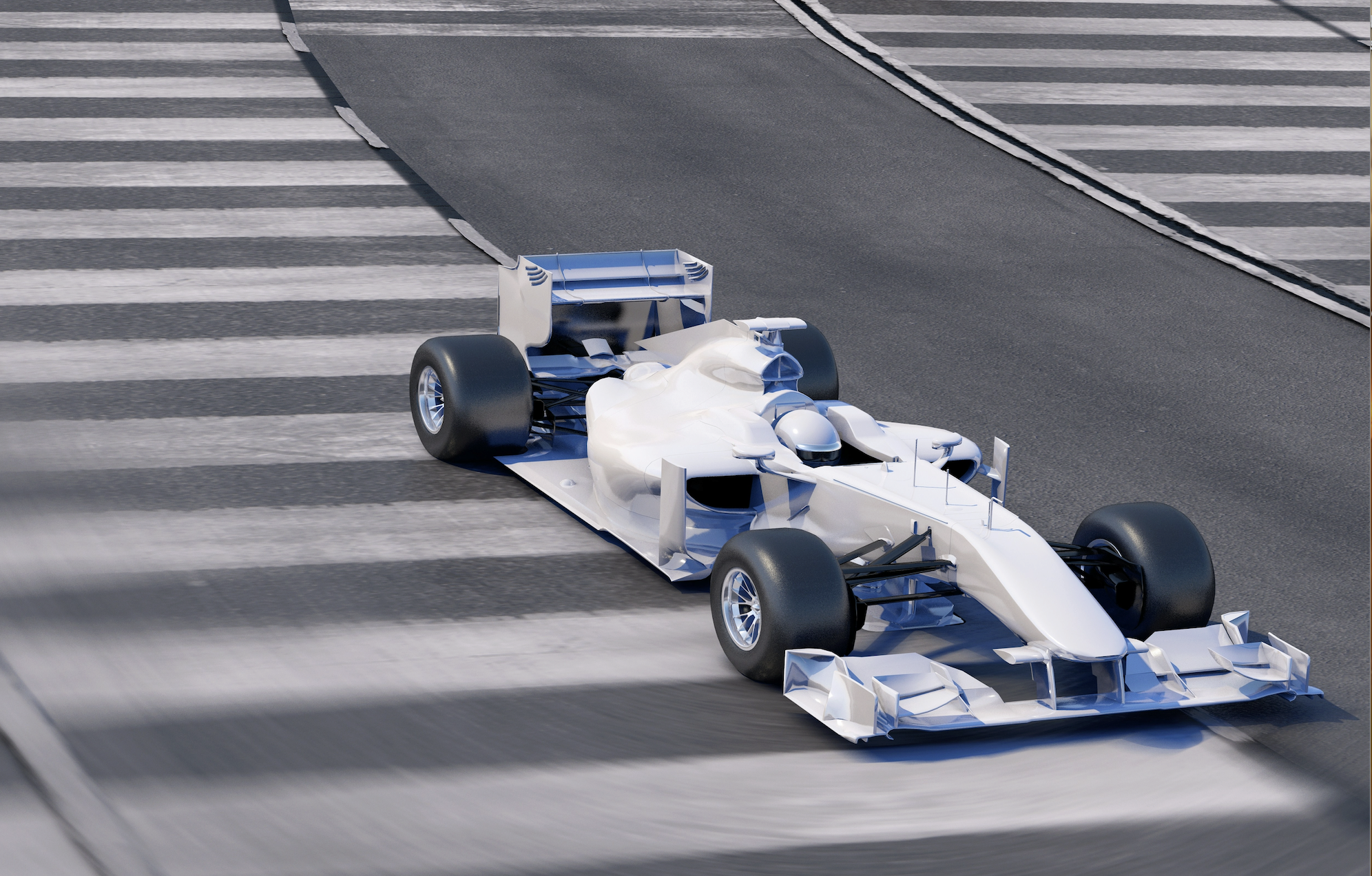
Growing up in Korea, I struggled against odds to nurture my unlikely love of baseball. I’d gather friends for pickup games in the city of Icheon’s only baseball field, relentlessly practicing my pitching, batting, and running to one day fulfill my childhood dream of becoming a Major League player.
Soon, however, as I watched new players get their big breaks, I began fantasizing about becoming an MLB scout. Could I predict who would become the next MVP? Younger players seemed like better bets, for starters. I felt confident when one of my top picks, 28-year-old Eric Hosmer, signed an eight-year, $144 million contract with the San Diego Padres in 2018 — but disappointed as he performed poorly, retiring in 2024.
I felt totally surprised when 38-year-old Nelson Cruz signed a one-year, $14 million contract with the Missouri Twins in 2019 — and even more so to watch him play well into his forties. Okay, so the business of predicting success is pretty complicated. Youth isn’t everything. I wanted to know more.
Computer Science Contagion
So, it was a dream to explore questions like these — and learn the tools of an MLB scout — in Wharton School Professor Adi Wyner’s five-week Moneyball FLEX program. It would have been a dream to attend in person on the University of Pennsylvania’s campus. But the online program suited my needs perfectly, as it allowed me to maintain my tough schedule of training for the 2025 World Lacrosse Men’s U20 Championship, while also working on data science research and projects. (BTW, lacrosse is a growing sport in Korea, and I’m excited to report that we’ll be hosting the championship here on Jeju Island this August.)
And even if I didn’t get to finally meet Professor Wyner in person (I’ve followed his Knowledge@Wharton videos and podcasts since 2020), his enthusiasm for sports and analytics was truly contagious, making me excited for each new lesson, even at the end of a long day when my class met.
The best part of the program, however, was being able to take all the data science and computer science techniques I’d learned to investigate what makes a player great. For my final project, I teamed up with three other high school students from across the world to determine how much age matters in athletics.
Sports Analyst in the Making
We decided to analyze the debut age of F1 Motorsports drivers, rather than baseball players. I had an F1 fanatic in my group, and on teams you sometimes have to compromise. We saw a similar trend that I’d seen in Major League. Some F1 drivers, like Sir Lewis Hamilton, achieve great success after debuting at just 22 years old. For others, however, youth doesn’t seem to be an advantage. Sébastien Buemi, for instance, also debuted at 22, but only competed for three seasons before retiring due to a tough losing streak.
Should he have waited before entering the race? Using charts and numerical analysis techniques in the programming language R, our team analyzed 860 drivers in an attempt to find an optimal F1 debut age. To our surprise, we couldn’t find one. Age wasn’t a significant factor after all.
Even if we didn’t crack the code for F1 success, I still found the project — and the process –incredibly satisfying. Learning and applying real-world data science techniques, I felt closer to achieving my dream of becoming an MLB scout or manager. Of course, to get there I still have a long road ahead. But I’ve started by using what I’ve learned in Moneyball FLEX to analyze baseball players’ debut ages. Have I landed on the magic number? Sports analysts don’t share their secrets.
Sewon L. is 17 and an 11th grader at St. Johnsbury Academy Jeju. Jeju Island is Korea’s largest and southernmost island. Sewon attended Wharton Global Youth’s online Moneyball Flex program, an introduction to statistics and coding, in 2024.