A Data Project Explores Gun Ownership in New York City
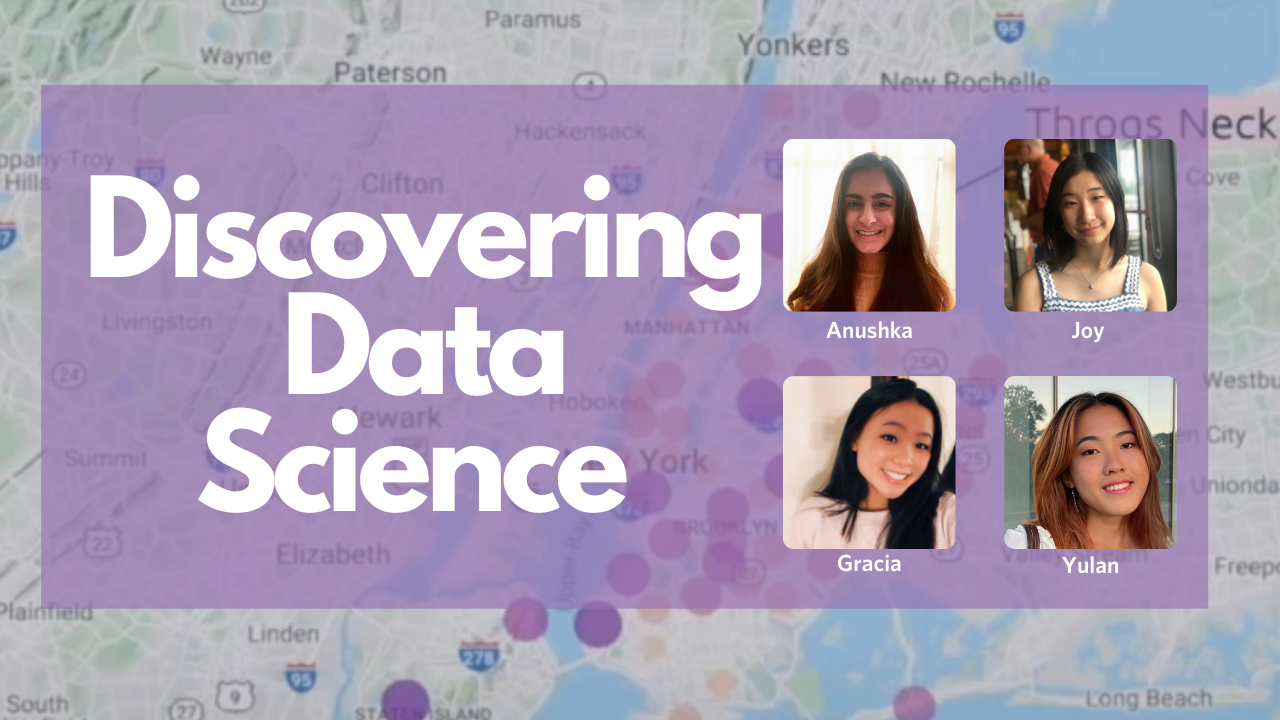
It all started in the summer of 2021, when a group of high school students from across the U.S. attended the Wharton Data Science Academy, a then-remote Wharton Global Youth program led by statistics professor Linda Zhao.
For their final project, the group of five chose to collect and analyze data on gun ownership, resulting in data-driven research on “The Demographic Effects on Gun Ownership in New York City.”
The topic struck a chord with this group of socially-minded youth. “Gun violence is a dangerous and alarming issue that has been growing exponentially over the past couple of years across the U.S., and especially in New York City,” says Gracia Chen, a senior at Miramonte High School in California who likes using mathematical methods to analyze society and human behavior. “We explored gun ownership in New York City to gain a clearer understanding of how different demographic indicators impact whether or not someone chooses to own firearms. We studied New York City because of the high concentration of gun violence in that region.”
In February 2022, the four women on the Data Science Academy team presented their findings during the virtual Women in Data Science (WiDS) @ Penn Conference, hosted by the Wharton School and Penn Engineering. You can watch their video presentation below!
Meanwhile, here are some highlights from their adventures in data wrangling:
📊 Prepping the Numbers. To prepare and clean the data for the project, the Data Science team loaded in two datasets, the first on the number of handgun permits in New York City by zip code and the other on the demographics of the 59 community districts in New York City, filtered to reflect only the most recent data from 2019. The numbers were taken directly from the Citizen’s Committee for the Children of New York and the New York Police Department. “During the first few days we definitely faced more challenges, like finding proper datasets, merging the datasets, and cleaning the data,” notes Anushka Acharya, a junior at Amity Regional High School in Connecticut. “However, as we continued on, we were able to overcome these challenges, and move quicker with the project due to our growing understanding of data science.”
📊 Visualizing the Data. Next came some Exploratory Data Analysis, where the team created various graphic representations of the data to understand it better. These included a bubble map of the 59 community districts representing the number of handgun permits per 100,000 people, a bar graph showing the 10 community districts with the highest number of handgun permits, a bar graph of the 10 districts with the highest percentage of homeowners, and a scatter plot of handgun permits per 100,000 people versus the number of reported violent felonies for each community district.
“The bubble map was a challenge,” recalls Yulan Wang, a junior at Theodore Roosevelt High School in Ohio. “Due to the format of our gun permits data, we could not use a pre-made R-package to merge the coordinates (longitude and latitude) of the districts to make the map. We were on the verge of abandoning the bubble map idea until we came up with the solution to manually add in each of the 59 community district’s coordinates. I stayed on Zoom with Anushka while we divided the task of putting in the coordinates. I remember the excitement I felt when I shared the bubble map with my team members!”
📊 Doing the Analysis. The team started its data analysis with lasso regression, a technique where data values are shrunk toward a central point. “We used the lasso function to select the most significant variables in our data,” notes Joy An, a junior at Choate Rosemary Hall in Connecticut who appreciated applying her computational skills to analyze real-world problems. “Next, we created a linear regression model using the variables that were selected by lasso, and finally we used the Anova function to generate a significance value for each variable and perform backward selection to eliminate insignificant variables.” Anushka recalls what she describes as a memorable outcome. “My favorite insight from the data was the results of the lasso regression. We found that a person’s job and home status have a significant impact on whether or not they own a gun.”
They rounded off the analysis using random forest, an algorithm that builds decision trees resulting in more accurate predictions about the data. For this, Yulan spent hours sitting at the coffee shop reading an academic paper to understand how random forest works. “It’s easy to run a line of code and apply random forest to the data, but understanding the mechanism behind it is much more difficult,” she notes. “It also made me realize all the different ways data can be analyzed by applying various machine learning methods. When people think of data science, most would think it’s boring doing the same tests over different datasets. But data science can involve so much creativity.”
‘Data Science Can Help Us Better Our World’
The team’s data wrangling and analysis yielded some unique observations about the effects of demographics on gun ownership in New York City. For example, the community districts with the highest concentration of handgun permits are in Staten Island and the Bronx, which have a relatively low population density. The most populated New York City borough, Manhattan, does not appear on the top 10 list.
“It surprised all of us to see that the number of gun permits in the most populated district, Manhattan, was not more than the least populated districts like Throgs Neck in Staten Island, a family-oriented area with more homeowners. It’s interesting to think about the causes behind this — the age group of the people living there and city apartment restrictions on guns,” says Yulan.
The project also underscored the importance of identifying biases in data. For instance, gun ownership is not accurately reflected by gun permits, since some people have illegally owned guns. Also, this particular study only focused on handguns. Other types of guns may be affected more by other demographic factors.
This team of aspiring data scientists has a message for other teens about the power of data.
“Data science can help us better our world by studying issues that need to be addressed, like gun ownership and gun violence,” says Yulan, who is currently using Natural Language Processing methods to research Twitter public discourse on COVID vaccines as a way to understand conversations about the vaccine and provide information to public health policymakers. She hopes to pursue a career in cognitive science. “No matter what your interests are — whether that’s business, health care, law, psychology, or literature — data science can be utilized and implemented into your studies. Data science can reveal trends and insights that we can all use to make better decisions.”
Gracia would encourage high school students to take on a bigger data science project and explore their skills. “Personally, this gun ownership project was a fun experience for me,” she says, adding that this was her first time analyzing data on this scale. “I think that the group effort made it manageable, exciting, and created a more in-depth analysis than I could have done on my own.”
Anushka, who lately spends at least part of her days analyzing data to identify relationships between gene expression and chromatin accessibility, shares one final personal takeaway from her Data Academy experience: “Data science is an exciting field that anyone can and should be a part of.”
I would like to congratulate these ladies for their efforts and dedication to this project. Women in STEM are unstoppable!
This article is particularly noteworthy because of the prevalence of gun violence, especially in the United States. This is an issue every American has learned quite a bit about, and from someone who leads a technology/STEM club at their school, the approach of using Data Science and Statistics to analyze this issue caught my attention. The conductors of this project used Data Science and Statistics, along with their savvy technology skills, to shed light on the factors affecting licensed handgun ownership in New York City. These scientists provide an excellent example of how Data Science can be utilized in order to have a deeper understanding of the problems that afflict our society.
In the United States, we face the horrific, and sadly common, issue of mass shootings. They have deeply divided the nation, with Americans looking to lawmakers for change. The nature of this article reminded me of a Supreme Court case I have studied, McDonald v. Chicago, in which Chicago was sued for preventing citizens from obtaining a permit for handguns, even for self defense, in an incredibly dangerous city such as Chicago.This, McDonald’s side argued, violated citizens’ Second Amendment right to bear arms. The reason for this ban was simple: a severe amount of gun violence. However, this did not effectively become the solution to this gun issue because there are many illegally owned guns that the government was not aware of and did not account for. This article further reminds me of this case when the author wrote, “…gun ownership is not accurately reflected by gun permits, since some people have illegally owned guns.” This line is so utterly important because it reflects the necessity of considering ALL variables in a scenario. In relation to the case, the ban could not prevent a significant amount of gun violence in Chicago due to the issue of illegally obtained guns. The women who successfully analyzed the data on handguns in New York City effectively accounted for all biases, and crunched the numbers. Their hard work can help the government of New York City, and lawmakers throughout the country to understand how Data Science can be utilized when trying to solve the issue of mass shootings and understand the demographics behind gun ownership.
According to the article, “Data Science Can Help Us Better Our World,” which I couldn’t agree with more. The beauty of data science is that the truth can be uncovered, as long as you are aware of notable biases, and anything that could affect your numbers. Numbers are a universal language. They connect to everyone and everything. There’s no escaping ending up in someone’s statistical analysis!
Talia, I appreciate your thoughtful opinion on gun violence and ownership problems in the United States, arguing that banning gun ownership now is too late to prevent escalating gun violence, as civilians in the States already own 400 million firearms, according to recent research from the National Public Radio. Currently, gun violence might be the most pressing issue in the United States because of the drastically increased amount of gun violence including the massacre that happened in Robb Elementary School, Uvalde, Texas. Like you, I also am aware of people around me, who bought handguns for “self-defense” purposes to protect themselves or their families from criminals or home invaders.
While I agree with your opinion that banning gun ownership now cannot stop people from using firearms that they have already bought, I still believe that further gun control measures should be implemented to put an end to our society mourning for the loss of innocent people dying from the irresponsible usage of firearms. Specifically, you have pointed out that the current law that restricts people from carrying firearms in the public environment has not done much in preventing gun violence, and therefore, is useless. However, I believe that the current law is less effective because the law itself is not strict enough to prohibit people from the irresponsible usage of dangerous firearms, portraying that the government needs to implement more rigorous gun control measures. For instance, the gun restriction policy of Texas, where the recent massacre happened, allows any individuals who are over 21 years old to buy and carry guns without any restrictions. Furthermore, Texas abolished the law that restricted people from having to provide their gun licenses to purchase a handgun. This means that now, people living in Texas do not have any legal restraints that check whether they are responsible enough to hold dangerous firearms that could possibly be used to kill other people only if they are over twenty-one. With these changes that overthrew many of the gun regulations, there has been a spike in gun-related casualties in Texas with more than 4,000 people dying because of shooting just in the year 2021 according to an article from the New York Times.
In addition, more severe problems arise from the lost and stolen guns. The number of guns reported stolen in the Lone Star State nearly doubled between 2007 and 2016, from 13,225 to at least 26,004, according to the National Crime Information Center, an FBI database used to track stolen property. Overall, at least 186,548 firearms were reported stolen in Texas over the last 10 years, which is the highest among any states in the nation. Even more, these statistics are believed to be undercounted as Texas does not mandate the reporting of missing weapons to police. What concerns people the most is that these lost guns are mostly used for gang-related activities, because unregistered firearms can not be tracked by the police, putting youths of the local communities in a position of risk.
Another argument you addressed was the tacit gun threats that pressure people to feel safe only if they have their own gun that they always carry to self-protect themselves from crimes. You gave us an example of this situation saying that restricting people from buying guns would not play much of a role in preventing gun violence because people still can buy lost or illegally traded guns in Chicago to protect themselves from criminal threats. . However, I think that because the government is not imposing a strict gun control policies, it is too easy for people to illegally obtain guns, which I believe will eventually cause more gun violence. Therefore, to break this unending negative cycle, I think the government should work on building a social mood that enables citizens to feel safe from any criminal activities and restoring abolished gun policies from the past to decrease gun violence.
In conclusion, I disagree with your opinion that efforts to increase gun restrictions in the United States are futile. I believe that there are practical benefits to restricting people from owning guns. The recent massacre in Uvalde Texas has proven that it is alarmingly easy for people to buy and openly carry a gun now in the United States. Restricting guns from people can reduce gun violence because it will provide a safer environment for people where dangerous firearms could be removed from the hands of irresponsible individuals who are exploiting the right to ‘self-defense’ as a means to harm others.
Talia, I appreciate the insight you shared about gun ownership and gun violence in the United States. You bring up some excellent points, such as how using data science and statistics is an effective way to analyze this issue. The McDonald v. Chicago case you mentioned is a perfect example of why data science is important to recognize all variables and biases that may alter the results of a study. You also noted that banning gun ownership in Chicago was a futile attempt to suppress the increase in gun violence, due to “the issue of illegally owned guns.” I agree that the ban was ineffective to prevent gun violence, and I would like to deliver my own thoughts surrounding this case and how more drastic measures can be implemented for a safer America.
Although it’s true that banning the ownership of guns in households will not get rid of guns that people may have already purchased in the past, I still believe that implementing further gun ownership restrictions may help prevent the ownership of new firearms and therefore, reduce the amount of guns overall in American households. Chicago’s law banning the possession of firearms in Chicago was ineffective because of the existence of illegal guns. However, if the law is not effective enough to ensure citizens’ safety, then there should be more rigorous laws in place to prevent irresponsible people from being in the possession of firearms, to decrease the number of said illegal guns. If the current law isn’t enough, then it simply means that there is a need for tighter regulations.
Another point you made was that because of the prevalence of gun violence, Chicagoans felt pressured to carry firearms in their own home in case they needed it for self-defense. Again, I believe that if there were stricter gun policies, it would make it more difficult to illegally obtain guns, which will decrease the need for people to own guns for self-defense. The government should focus on stricter laws to reduce ownership of guns overall, leading to a safer atmosphere. Once this atmosphere is acquired, people will feel less obliged to own guns for self-defense, and altogether, gun violence may come to a screeching halt.
A perfect example of this positive cycle can be seen in South Korea, a country with extremely strict gun regulations. People must first obtain a license and even with a license, they may only purchase air rifles or hunting guns. Furthermore, when they are not in use, firearms are kept at local police stations. The effects of these laws are clear: the rate of firearm violence in Korea is extremely low, with rates ranging from 0.01 to 0.02 per 100,000 people. In other countries with similar gun control laws, such as Japan and Singapore, the firearm crime rate is similarly low. When it comes to gun violence, the U.S. could learn a thing or two from these countries. If there are more regulations on gun ownership, then not only would the number of irresponsible individuals with guns decrease, but less guns will be floating around in households because there will be less of a need for self-defense. You’re right, it’s hard to control those that obtain guns illegally, but we can start by lessening the amount of people that, under the guise of “self-defense,” will purchase weapons to harm others. Therefore, I argue that placing stricter gun laws similar to those in the countries mentioned above is the best way to ensure people’s safety and decrease the number of mass shootings and gun-related violence.
I believe that there is no harm that would come out of making it harder for people to own guns. There is a reason why the U.S. has high firearm-related crime rates when compared with other countries: because it is alarmingly easy to own a gun. Making it harder for people to own firearms will increase safety because it removes guns from the grasp of irresponsible owners and gets rid of the need for people to purchase guns as a form of self-defense.
My first thought when reading through this article was, what about the starter pistols for horse races? Surely, those are being tallied up in the count as well, right?
My second thought? There are so many takeaways from Gracia Chen, Anushka Acharya, Yulan Wang, Joy An, and Sidarth Krishna’s data project that stem beyond from what was presented. In particular, one interesting finding mentioned in the Women in Data Science presentation video but not in the article is that there seemed to be fewer violent felonies when increased rates of obtaining gun permits were experienced.
Overall, their work not only brings up strong points about the demographics of gun ownership in New York City, but it also underscores the importance of data analyzing and its overarching relevance to everyone. Such a process is especially crucial for drafting and implementing new pieces of legislation with regard to gun control; demographics and other data must be taken into consideration beforehand to understand the situatuion at hand, and analysis on death certificate data like sucide and homicide rates ought be collected afterward to determine the effects.
However, too often, statistics like these are taken at face value, stripped from their emotional baggage and what they truly stand for. Sure, many people might know that there have been more than 250 mass shootings in the United States just these past six months, but it’s hard to conceptualize this data into real people: children, toddlers even, a best friend who someone shared secrets with, secrets that will die along with them, mothers, teachers, the list goes on and on. Even those wounded by shootings will be left with lifelong physical and emotional scars that can never be healed.
The fact is, we know the numbers and sometimes we recognize the names, but we fail to put two and two together when it comes to faces and the actual identities of these victims. As someone who was a part of a similar data project that conducted research and drew conclusions about Lake Mead’s falling water levels, I was also under the same impression that data was nothing more than just ancient history, organized for all of us to see in neat, organized little rows and columns. However, it doesn’t take much to change that perspective.
Where our cross-country team once waded through ankle-deep rivers of mud, fought for our places in the strung-out line of rosy-cheeked runners, and celebrated with their team by raising bottles of Gatorade in cheers: Oxford. That day, I’d even shared Strava profiles with a senior.
The boarding school that I went to was less than a half-hour drive away. It only made sense, then, that the news about the Oxford High School shooting was turned on almost every laptop, television, and smartphone alike that afternoon; school was immediately canceled with a ding! notification in our emails, and legions of worried parents proceeded to grapple for the tiniest spaces in between the growing line of cars. Funny enough, it was just like we did at the Oxford meet a couple of weeks ago.
These students, coaches, teachers, and staff who were scarred by this shooting were friends and family to people that I knew. No longer did these shootings feel like a simple showing of statistics, where the public attention directed toward these tragedies was determined simply by the head count of casualties, but this event dealt a neccessary reminder that real, innocent lives were lost. Just one undue death by gun violence would set off a cascade effect of devastation for dozens of loved ones. If you would walk into any one of the classrooms at my school, you’d find so many empty chairs for days on end, motivated dually by grief and also fear.
Though having personal experience with gun violence isn’t something I’d wish even on my worst enemies, would the average person look at the news and see the number of deaths from a shooting as any different from a weather report?
What I appreciate most about Gracia, Anushka, Yulan, Joy, and Sidarth’s work is that it showcases how teenagers, along with the general public, have the resources available to take incentive and examine data trends at their very fingertips. After all, each and every newly-drawn conclusion contributes to a more holistic understanding of the bigger picture of arms violence and how we can better promote safety when it comes to firearms. In addition, spending time mulling over all of the different factors and variables really builds a deeper, more meaningful connection when compared to just sifting through raw data.
We got the kick-start we needed from this presentation on New York City; how about we tackle the entirety of the United States next?
Hey Eric,
Firstly, don’t forget about the starter pistols used for track & field races, or the ones fired during swim meets!
All jokes aside though, thanks for your insightful comment. Like you mentioned, the research done by Gracia Chen, Anushka Acharya, Yulan Wang, Joy An, and Sidarth Krishna was genuinely eye-opening, and as a New Yorker myself, it was quite interesting to observe the statistical distribution of gun permits throughout the city. At first, I was a bit surprised to see the absence of some historically dangerous NYC neighborhoods on the bubble map, such as Brownsville and Bedford, but I realized that gun ownership in these areas is often unauthorized, with illegally owned guns being the norm. I appreciated how the researchers acknowledged this potential bias in their study, also mentioning that the particular study they conducted only focused on handguns.
As we continue to move into an increasingly technology-driven world, it is evident that big data will become a catalyst for addressing major issues in our society. From public health policymakers to sports team managers to non-profit CEOs aiming to alleviate the global refugee crisis, everyone is in thrall to the big data juggernaut, relying on it to accurately portray the situation at hand and devise strategic solutions to address them. As Yulan put it, “data science can reveal trends and insights that we can all use to make better decisions.”
But as you eloquently mentioned in your response, there is a genuine and growing fear that big data will have a dehumanizing effect on our society. As Joseph Stalin may or may not have once stated, “the death of one man is a tragedy; the death of millions is a statistic.” This quote perfectly summarizes the phenomenon of extension neglect, the idea that as human beings, we don’t cope well with escalating numbers; the loss of hundreds, thousands, even millions of lives tends to be viewed as a statistical reality for many of us, rather than a personal one.
I am so sorry to hear about your personal connection to the Oxford High School shooting. Though it is difficult for me to admit, my initial response when I saw the tragedy on the news was not one of condolence, as it should have been. Instead, it was one of resignation. Though the number of deaths from the shooting, four, was far from mind-boggling, I subconsciously added the number to the 17 from the Stoneman Douglas High School shooting, then to the 10 from the Santa Fe High School shooting, and the 28 from the Sandy Hook Elementary School shooting, until everything became one conglomerated blob of numbness. Before the Oxford High School shooting took place, did you have a similar response to the shootings as I did?
Like you, I have found that tragedies like the aforementioned school shootings hit closer to home when they impact those in our actual lives. If you recall, at the height of the coronavirus pandemic, Anti-Asian hate crimes also hit a peak. It was during this time that the mom of one of my closest Chinese friends fell victim to one of these racially motivated hate crimes. While out for grocery shopping just a few blocks outside her home in Chinatown, she was brutally assaulted. The assailant tripped her to the concrete floor and proceeded to kick her multiple times on the side of her face. The sight of her in a hospital bed, her head wrapped in bandages to ease the swelling and blood, her hands grasping her son’s, was stuck in my mind for days. To me, the memory was so much more meaningful than the statistics I saw on the news or on social media. To me, the one mattered more than the two hundred and thirty three.
Many people refer to this kind of experience as an example of the empathy gap, a phenomenon that occurs when numbers get so big we cannot truly fathom the real people behind them. Feelings are certainly less quantifiable than data points are, but the value of empathy, whether we can measure it or not, should not be ignored. How can we ensure that big data becomes representative of the genuine faces and stories that compose them? I don’t have an answer yet, but hopefully one day we will reach a point where we will achieve it, together.
Hey Taee and Eric,
Thank you to both of you for your insightful comments. You both bring up really important aspects of the rising number of shootings in New York City: the unauthorized possession of guns and the growing number of shootings used for increasingly unfortunate reasons.
It’s heartbreaking to see the city I was born and raised in be used in a study for our “high concentration of gun violence,” but ultimately, nothing is worse than the drop in my stomach I had April 27th, this year. My neighbor had texted me “stay safe, two people are hospitalized from a shooting outside of the preschool.” She’s a friend of mine and we always commute home from school together. The preschool she was talking about was the one that we passed every single weekday, the one that was an 8 minute walk from our houses, the one that we would’ve been outside of at the time of the shooting if not for the half day we had.
For the next couple days, I religiously checked the news coverage on the shooting. Turns out the people hospitalized were two teenagers: one 14 and the other 18. They had been shot by their peers at my local high school over gang related activities.
I couldn’t quite grasp how close I was to that shooting. Even though I live in a city known for shootings and quite literally hear news about schools and subways getting shot up in my borough, that’s all they ever really want: news. Numbers and statistics. But seeing kids my age on the streets I walk, makes me realize each and every one victim is a human with a life as vivd as mine.
Perhaps what’s more powerful than these statistics that we hear all the time is simply the fact that the three of us all had stories of our own that we thought of. Yet that’s the irony, the most powerful and eye opening way to see gun violence is what we want to avoid. We shouldn’t have live in a world where someone needs to have personal ties to gun violence to realize how serious of an issue it is.
This is precisely why I am so interested in the way these wome are analyzing data. By analyzing the neighborhoods and concluding more about gun violence in communities, we manage to humanize the data in a way that is more engaging to people without it being heartwrenching.
Hello Eric, I appreciate your anecdotal experience of gun violence, providing an insight on the statistics and ways to improve them. Your artistic way of writing, truly made me sympathize with your story, raising an alarm that gun violence should be put to an end and that these tragic massacres should be viewed beyond numbers and graphs. Although I have not experienced gun violence and have not lived in a country that legally allows people to own a gun, when I read news and reports about gun violence, I questioned myself why would the government allow people to own a gun when death and destruction caused by it are exponentially increasing, leaving an unerasable trace of trauma to the hearts of the people.
I agree with your comment that statistics should be more emotionally captivating in a way that could visualize the ramifications of the escalating gun violence in our society and address the lives of the individual victim in detail. Although data analysis can provide insights to incorrigible pro-gun groups, that supports unrestricted gun ownderships as a tool for ‘self- defense,’ the data and statistics do not show the real-world emotional implications of how innocent lives were lost. For example, the tragic death of George Floyd was able to raise a great amount of public interest, because the media focused on appealing to the emotions of the people. Specifically, they portrayed the personal background of George Floyd and the terrifying story of how he was killed by the police, and did not concentrate on explaining numbers and statistics related to black people being killed by the police. Therefore, I agree with your argument that statistics and data have a weakness in bringing strong public attention to the individual lives of the people.
Still, I believe that there are reasons why statistics and data are presented in a way that disregards portraying human’s real life. By removing pathos when gathering, analyzing, and visualizing data, they can provide an objective evidence for the individuals to create unbiased judgment based on numbers and statistics. Because of this feature, data can be extremely helpful when assessing what is the most pressing problem that needs to be fixed in our global society. For example, according to the research from United Nations and Amnesty International, if you compare the number of people around the world dying due to starvation and the number of people dying due to gun violence, the number of people who died from starvation is 50 times greater than the people who died because of gun violence. So, if we view the two different problems from a utilitarian perspective, ignoring any of the feelings and evaluating them just by numbers, the world should focus on helping millions of people who are at risk of starving to death to improve the lives of as many people as possible. However, if data and statistics are directed towards appealing to people’s emotions, they may create biases in people’s minds when evaluating and determining what the most pressing issue is in our current society. When we are assessing the severity of the problem, do you still think we would be able to be objective when numbers are affected by emotions?
Please don’t get me wrong. I am not trying to say we should not care about the lost lives of the innocent or mourn for those who have been sacrificed by gun violence. I am a strong advocate of pro-gun control, who cannot even fathom how it would be like to be that little girl — that brave little girl in Uvalde, smearing the blood off her murdered friend’s body onto her own face to pretend she was dead in order to stay alive; and how what emotions she would feel when she walk down the hallway of any school after surviving from the threat of being killed.
However, I also want to alarm that although data about our societies are created based on the real lives of the people, focusing too much on representing the individual stories may hinder numbers and statistics from truly demonstrating their greatest strength: providing factual information that enables people to create unbiased interpretation.
Dear Eric,
First of all, I appreciate your thoughtful response. Yes, I agree that gun crime rates in the States are escalating higher and higher, which can be inferred by looking at the data chart for New York City in the original article. I also agree that criminal offenses and social controversies associated with gun violence in the States are becoming more severe, and that gun control should be strengthened. However, I believe that one’s right to bear firearms should not be entirely eliminated from the Second Amendment of the United States Constitution.
Certainly, It is unfortunate to hear about the tragic events on gun violence that happened due to the gun laws that are too weak to prevent irresponsible usages of firearms. Many mass shootings, such as the recent incident at Robb Elementary School in the small town in Texas, are happening broadly around the United States. I am sorry to hear that you also have gone through a similar unfortunate event. I too have a close friend, William, who has not only experienced being threatened by a gun but also lost his stepbrother due to a school shooting. His stepbrother has attended a public school unlike William who went to a junior boarding school at the moment. Will still remembered every single detail of the day, such as the weather and the exact time when his innocent step brother died brutally in a massacre. The event left a lasting scar in the minds of William and many other families who have lost their children, brothers, or sisters. Yet, it is stunning to think that these shootings — these ruthless attacks on innocent children — aren’t continuously happening in our society. .
However, I still believe that the right of the people to bear firearms should not be infringed as there are cases where gun saved the lives of the people when it was used in the right way. Specifically, there are people in real life who have used legally owned weapons for self-defense purposes. For instance, in the summer of 2012, Ms. Jessica McBraven defended herself and her newborn baby from two home intruders., in which she killed one of the intruders with her shotgun. Her story is one of the exemplary cases that portrays how a gun can save innocent people’s lives when it is used in the right hands. Another famous story of correct gun usage is how Mr. Steven Anderson protected himself and his dog from a 700 pound grizzly. As Mr. Anderson saw a grizzly bear approach his dog, he got his rifle and shot it into the sky, which scared the grizzly away. These real life stories prove why gun ownership rights shouldn’t be completely removed .
To conclude, more gun control laws should be enacted to prevent firearms being used in the wrong hands and to reduce gun violence; however, the rights to own a gun should not be completely removed. While there are people who exploit the loopholes in the current gun law, there are also responsible people who use guns in the right situation at the right time to protect themselves and their loved ones. It is unfortunate to hear about all the innocent victims who have lost their lives due to gun violence,happening all around the United States, but this does not necessitate exterminating people’s right to possess tools to protect themselves from external threats and dangers. What we truly need to work on is coming up with stricter gun control laws that forces individuals to go through a more careful and detailed checking process when purchasing a gun.
YOO, I respect your perspective on enhancing gun regulation laws. Gun usage is considered one of the biggest threats to the citizens of the United States, and countless lives are being taken by shooting every year. However, although I aspire to your opinion on permitting firearms, I have a different point of view on the issue.
You mentioned the second amendment of the US Constitution as a reason to back up your argument on the right to bear firearms. However, the second amendment is considered invalid by many scholars these days. The second amendment was established in 1791 when George Washington was in office. The most common guns back then were muskets and flintlock pistols. These weapons were highly inaccurate at a certain distance and took a long time to reload. This was why the citizens did not identify firearms as a huge threat. However, guns used nowadays are considerably deadlier than those in the past. It is superior to the guns used more than 2 centuries ago in almost every aspect, enabling severe incidents such as mass shootings. Moreover, the language of the second amendment is ambiguous and can be interpreted in multiple ways. As it can be inferred by the term ‘militia’, the second amendment mainly addresses the right to bear arms in a collective term of rights, not the individual rights to do so. There has been an ongoing debate about the translation of the second amendment. Since the meaning isn’t precise, the sentence can not be used as valid evidence for the permission of guns.
Nevertheless, I agree with your statement that guns can be used as a means of self-defense. Carrying a gun can be recognized as a sign of hostility. Although it can threaten the citizens, it can do the same for the offenders. The awareness that their target is armed can reduce the likelihood of an attack, preventing potential crimes. Additionally, carrying a firearm can protect individuals in immediate situations, buying time until law enforcement officers arrive. One example of defensive gun use is the case of the Trolley Square shooting back in 2007. The incident took place in a shopping mall in Salt Lake City, Utah. Armed with several firearms, the suspect opened fire toward passersby, resulting in multiple casualties. Luckily, a police officer named Kenneth Hammned to be in the mall at the time of the incident and overpowered the shooter with his handgun. The presence of firearms minimized the damage of the case which could have been a much more fatal one. This provides empirical evidence that guns can secure the well-being of citizens.
However, despite the benefits of guns, strict laws that can regulate gun usage are still in need. First of all, a license and other paper that can prove one’s credibility is needed. Only a dozen out of 50 states need permission when purchasing a handgun, and only three require permits to buy rifles and shotguns. If more paperwork isn’t needed, firearms would be more likely to end up in the wrong hands. Utilizing technology could also be a good way to mitigate gun violence. For instance, GPS systems can be installed in guns, only allowing them to be fired inside the licensed area. Also, biometric technology can be used so that strangers with bad intentions or children can not fire the weapon.
The effect of guns is like two sides of a coin. The outcomes depend on the control over them. If firearms are supervised with strict laws and secured with safety mechanisms, they can be used as a powerful means of self-defense. However, with the absence of certain regulations, it will do the very opposite, resulting in great tragedies. An American comedian Chris Rock once said, “If a bullet cost five thousand dollars, there would be no more innocent bystanders.” As the analogy goes, I hope all gun owners use their weapons wisely, so that every shot made is worth the value.
Gun violence is an incredibly American issue. Although gun violence has been prominently disputed over the past decades, the recent school shooting in Uvalde, Texas reignited the debate over access to firearms in the United States. As a student, the idea of a not being able to learn without the threat of imminent death is concerning to say the least. Many students, among all others, call for increased laws and caveats in regards to purchasing a gun for better gun safety. Red flag laws and universal background checks are among the main solutions proposed to mitigate the detrimental effects of guns, but how can all parties be satisfied? How can the Second Amendment rights of Americans be protected to those who value their Second Amendment rights? The relevance of the ownership of guns is currently seen as a subjective matter; however, the dispute of the ownership of guns is also very objective. When people ascribe subjectivity to guns laws, it fosters this sense of irreversibility and difficulty in regards to finding an effective solution. By looking at the data statistics behind gun violence, the issue of gun violence can be looked at more objectively than subjectively, advancing towards an effective solution. It is important to view polar issues such as gun rights as something that can reach a consensus. I feel that, through the establishment of correct facts and effective civil discourse, we can find that we agree on more than we disagree. By making known the accurate facts behind gun laws and gun ownership, the conflict of gun rights can be resolved objectively.
Also, I just had to commend these amazing women on their commitment to this project, overcoming roadblocks and forging the path towards solving pressing issues. This taught me a great deal!!
I’m constantly blown away by the level of innovation and creativity of my fellow high schoolers, and it’s certainly the case with this amazing group of dedicated and talented ladies and their data project regarding gun ownership in NYC. They took into account the number of handgun permits in NYC and demographic indicators (e.g. a person’s job and their home status) to explore the relationship between them.
Gun violence, no doubt, has become a pivotal issue in the United States, especially in the context of school shootings, which have already racked up to 27 just in 2022. It’s utterly heartbreaking to be on the way to fourth-period English class and see headlines of gun violence at schools fill my social media feeds. The current gun violence epidemic that is plaguing the Big Apple is no feat to be ignored. This data project demonstrates how we can take data science and place it into a variety of fields ranging from business, law, and health care as it helps reveal trends to help us fit the pieces to complete the bigger picture. The specifics that this group of young women targeted can be used to help local NYC government officials and policymakers better address the prevalent issue of mass shootings. These statistics go a long way in building a city that promotes the safety of its people when it comes to guns.
My one standing issue with data projects is that many times when people see statistics like the ones presented, they take it for just numbers. The number of people that own guns, and the number of gun violence-related deaths, these numbers are just grazed by and often many gun-related incidents aren’t nearly as covered as if the numbers don’t reach a threshold. This completely dehumanizes these incidents down to mere digits rather than real-life stories and tragedies that happened. Unfortunately, this comes as a result of living in an increasingly computerized world, which turns everything into data and numbers and loses the humanity aspect. We have come to a point where we are so desensitized to tragedies like this that we allow for real human lives and their stories to be thrown to the curb. As I mentioned previously, my news feeds get filled whenever gun violence tragedies occur, but the headlines every time, without fail, emphasize the numbers, rather than the people who were involved. An addition, I would make to this gun ownership data project is to have a function to click into the various NYC neighborhoods and attach news articles regarding gun violence. This way it can report the gun ownership statistics alongside the stories so that the human aspect is not lost. One step further could even be highlighting the victims that were involved in these incidents, because, unfortunately, many times when gun violence crimes occur, the names of the assailants are plastered in the news, while the names of the victims are barely even mentioned. We have to make efforts to depict the entire story and showcase the very realness and humanness of these statistics. While there may not be news articles written about the victims, we have the opportunity to challenge this status quo of leaving victims and their families in the dark, while their assailants are on the front page of every news channel. This can include biographies and background information about the victims, and perhaps even interviews and/or comments from the victims (if possible), their families, and their friends to give back the humanity that hard numbers strip from them.
In their video presentation, next steps were mentioned for their data project, which I would like to echo. Expanding their project beyond NYC is crucial as different cities have different laws and regulations (open carry vs. concealed carry) regarding gun ownership. Furthermore, since gun violence is not just an issue that affects NYC, it would be beneficial to take into consideration other locations to have a better holistic understanding. This could help target not only regional policymaking but be brought onto a national level as well. Perhaps understanding this data can broaden our understanding of the underlying factors of gun violence, and then address the prevention infrastructure necessary to protect our country’s citizens from the gun violence epidemic.
Gun violence can be reduced by making people show identification before buying a firearm. This way guns never get into the hands of crazy people or people with ill intent to begin with.